AI
Predictive AI: Introducing Google Cloud Vertex AI and AutoML to Predict Booking Cancellations
Booking cancellations are a multifaceted challenge across the hospitality and travel industries, deeply impacting companies’ financial stability and operational efficiency. The average percentage of canceled reservations, across all sources, is currently 24%. Therefore, when you calculate your future revenue, there is one nasty surprise – you should always reduce your existing reservations by 24%.
Far from being merely unpredictable annoyances, these cancellations open the door to nuanced discussions about demand forecasting and customer behavior analysis. As AI providers, our job is to build a machine-learning model to help business owners better understand if the customer is going to honor the reservation or cancel it.
AutoML, in this regard, is like a crystal ball that doesn't just tackle large volumes of data but transforms it into strategic foresight. The true beauty of AutoML lies in its ability to democratize data science, enabling industry professionals – regardless of their technical expertise – to predict cancellation trends with remarkable accuracy. Moreover, this shift equips managers not only with the knowledge of when cancellations might spike but also why, and how different variables interact to increase the likelihood.
In one of our recent predictive AI projects, we implemented AutoML using Google Cloud Vertex AI to tackle booking cancellations. Software development extends beyond just predictions; we developed an intuitive app that centralizes these insights, offering real-time data to proactively mitigate the effects of cancellations. In this article, we share detailed insights into how we achieved this, showcasing the innovative methods and strategic applications that set our project apart.
The empty room challenge
In the hospitality industry, an empty room is a missed opportunity.
And statistics highlight a troubling trend: cancellation rates are climbing, with as many as 40% of bookings made with some channels being canceled before the stay commences. This suggests a shift towards more flexible travel planning, where commitments are viewed as tentative.
Understanding why cancellations happen is crucial for minimizing their impact. Seasonal variations are a significant factor, with peaks and troughs dictating booking behaviors. During the high season, hotels might experience fewer cancellations due to high demand, whereas the low season often sees spikes in cancellations due to unpredictable travel plans.
Moreover, customer behavior adds another layer of complexity, with last-minute changes often influenced by personal circumstances or better deals elsewhere.

A detailed analysis, supported by findings from Booking.com, sheds light on compelling patterns:
- The median lead time for cancellations made through online platforms stands at approximately 15 to 30 days before the check-in date.
- The timing of bookings also plays a crucial role in predicting cancellation likelihood. Bookings made well in advance are subject to higher cancellation rates due to the increased probability of changing plans.
- Online bookings make up almost 60% of all hotel bookings
- Couples cancel 66.7% of their bookings, significantly higher compared to families, who show a modest 11% cancellation rate.
These insights paint a clear picture of the challenges faced by the hospitality sector, leading to at least three critical outcomes:
- Revenue loss from the inability to resell canceled rooms.
- Higher costs from relying on distribution channels and marketing to fill vacancies.
- Reduced profit margins due to last-minute price reductions to resell rooms.
What do hotels traditionally do to predict and prepare for cancellations?
Typically, hotels predict and manage cancellations by analyzing historical booking patterns and enforcing strict cancellation policies. They examine past data for similar time frames to forecast cancellations and adjust operations, like modifying rates or intentionally overbooking. For example, a hotel might notice increased cancellations during the winter months in past years and might prepare by overbooking during these times to counteract anticipated losses. However, this approach has limitations, especially when unexpected events – such as new travel advisories or economic downturns—suddenly alter consumer behavior, revealing that these traditional methods may not fully capture or adapt to rapidly changing market dynamics.
Thus, hotels are now integrating advanced analytics and flexible strategies to better navigate the complexities of managing cancellations. These include:
- Advanced analytics and predictive AI to improve their prediction accuracy.
- More flexible cancellation policies for today's consumers who value flexibility.
- Proactive communication with guests about potential disruptions (e.g., adverse weather forecasts) and offering incentives for rebooking rather than canceling. For instance, offering a discount on a future stay or a free upgrade can entice guests to change their plans rather than cancel outright.
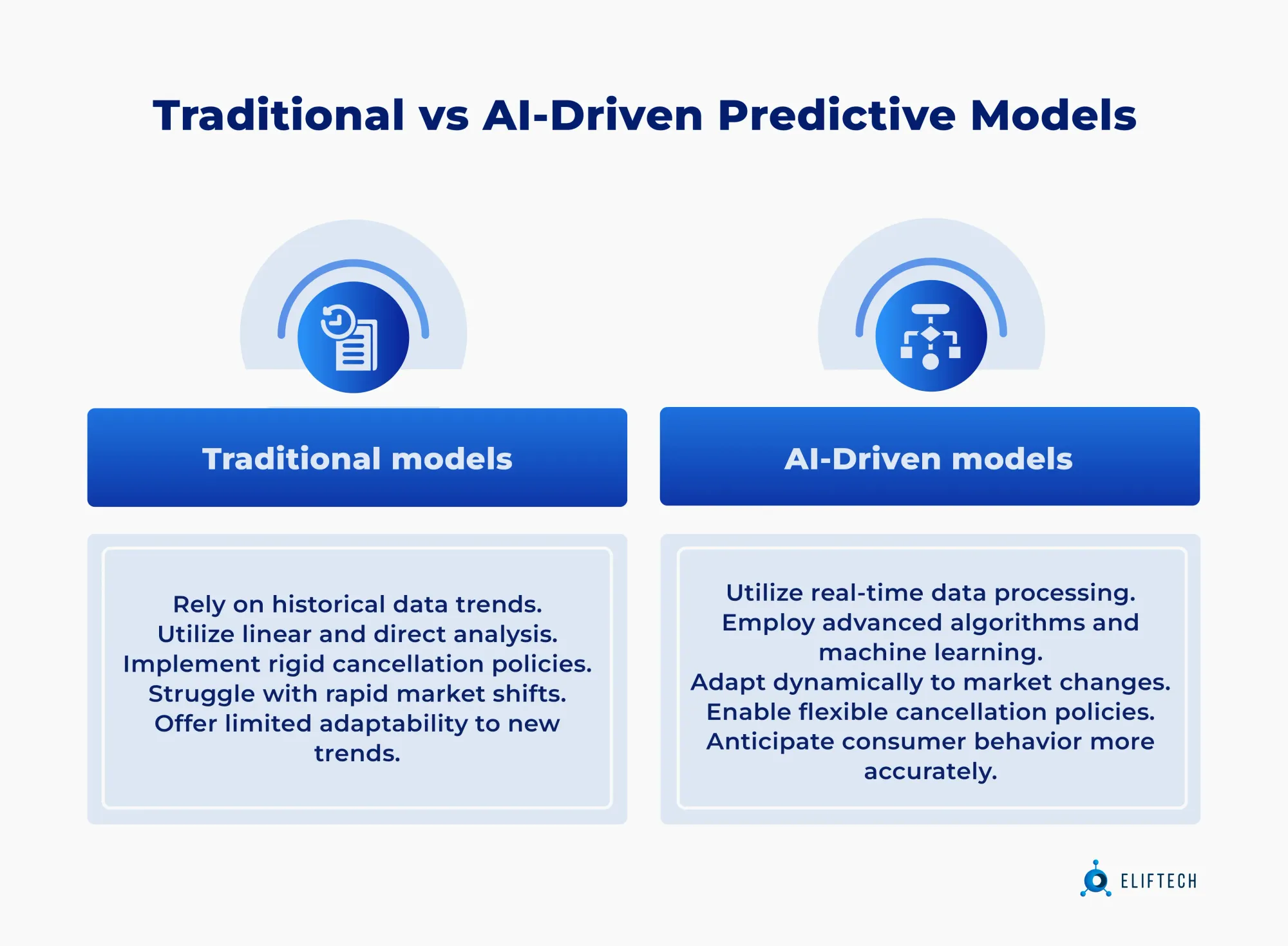
Traditional vs. predictive AI models
Dealing with the ups and downs of cancellation rates, hotels are looking for new solutions, stepping away from the old ways. We all know that AI makes everything smarter and enables hospitality and travel to take a step forward and not only analyze the past but also forecast future trends with a high degree of precision. From helping plan trips with chatbots to using smart models for better decisions, AI is changing how businesses work and how people travel.
In fact, studies like those from Statista show how quickly big travel names are picking up predictive AI software and how travelers are getting more interested in using AI to help plan their adventures.
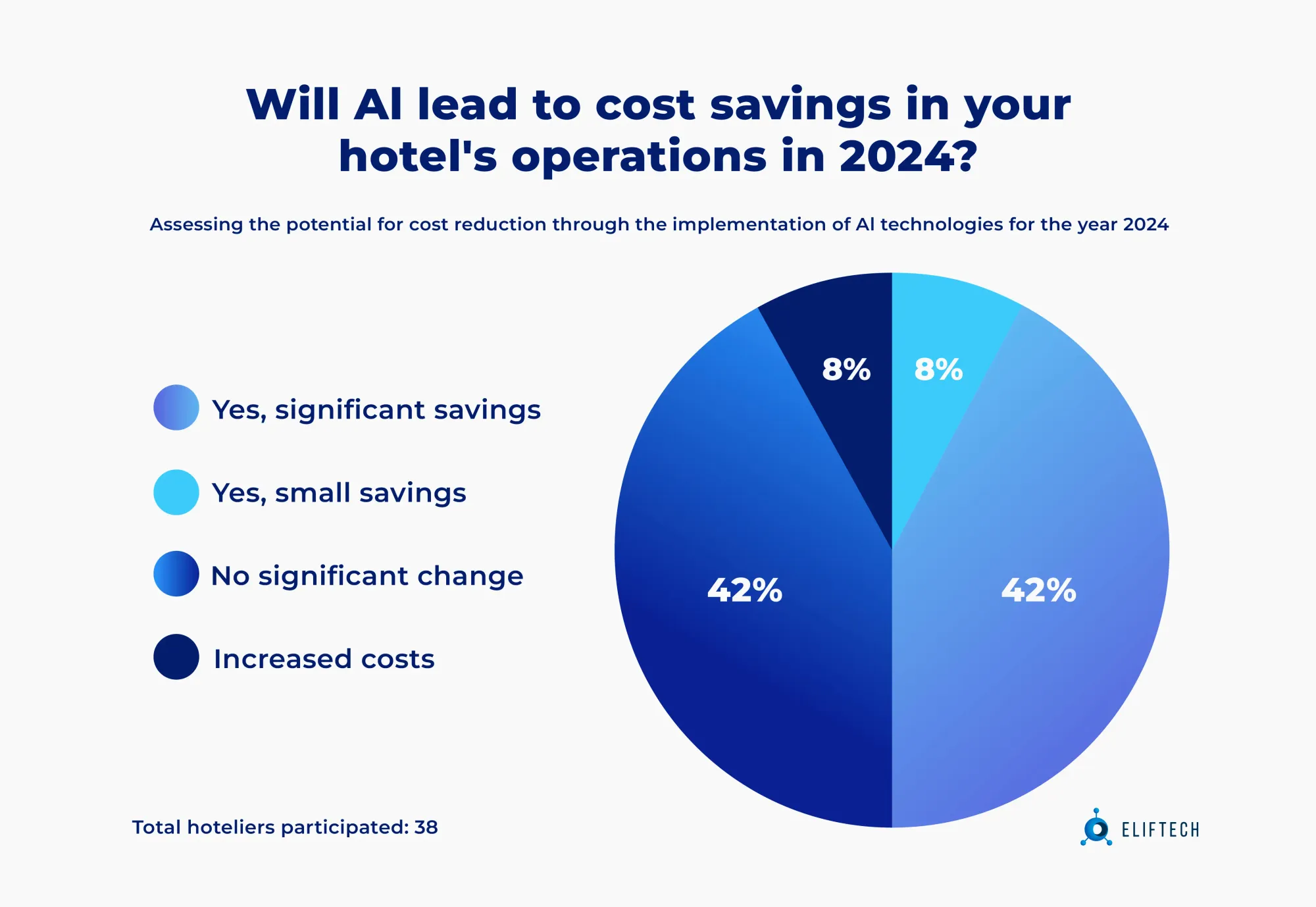
And what’s equally important is AI and automation can increase hotel revenues by at least 10% and cut costs by 15%.
But how exactly can predictive AI be applied to predict and reduce cancellation rates? There are several areas of influence.
Using ML to improve forecasting
Machine learning brings a nuanced understanding to the complex patterns of cancellations by sifting through vast amounts of data that encompass past booking histories, individual customer behaviors, seasonal variations, and market dynamics. This analysis not only forecasts when cancellations are more likely to occur but also identifies the probable reasons behind them, enabling hotels to tailor their inventory management and operational strategies accordingly.
Here are the typical datasets ML takes to predict hotel booking cancellations:
- Historical booking data: Records of past bookings, showing trends over time.
- Customer profiles: Information about customers, including repeat visit frequency and demographics.
- Cancellation histories: Patterns and circumstances under which past cancellations occurred.
- Pricing and promotions: Past pricing strategies and promotions, and their impact on bookings.
- Seasonal and event data: Local events, holidays, and seasonal trends affecting demand.
- Economic indicators: Broader economic conditions that might influence travel and booking decisions.
- Competitor data: Competitor pricing and availability, which may affect a customer's likelihood to cancel.
Bringing sentiment analysis to the game
AI can be employed to perform sentiment analysis on customer reviews and feedback, identifying potential causes of cancellations. AI can use NLP to analyze it whether through direct communication or social media platforms and gauge customers' mood and satisfaction levels, which can be predictive of their likelihood to cancel. Hotels can then address these issues proactively, improving service quality and reducing the likelihood of cancellations.
Enabling effective overbooking management
With predictive AI, hotels can intelligently manage overbooking by understanding the likelihood of cancellations and no-shows. For instance, by analyzing past booking trends, customer behavior, and even external factors like events or weather conditions, AI can predict that during a major local event, cancellations are less likely than usual. Instead of a hotel blindly applying a standard overbooking strategy – perhaps accepting 10 extra bookings for 100 available rooms – AI can refine this approach. It might suggest adjusting the overbooking limit to just 5 extra reservations, considering the lower anticipated cancellation rate. This precise adjustment ensures high occupancy levels without overstepping, thus reducing the risk and cost associated with overbooking.
Turning on automated rebooking
In the event of a cancellation, predictive AI systems can instantly look for similar profiles from the waiting list or reservation inquiries to fill up the vacant slot, minimizing revenue loss and improving the chances of occupancy. The AI assesses the needs and preferences of these guests against the canceled reservation details, such as room type and stay dates, to find a good fit.
The system takes into account both guest preferences and potential revenue opportunities, perhaps favoring profiles of guests who tend to book longer stays or spend more on hotel services. After finding the best match, the AI can automatically rebook the room and send out a confirmation, ensuring a smooth and efficient process.
Generative AI vs predictive AI: Introducing chatbots for effective communication
So, what about generative AI vs predictive AI? AI-powered chatbots can engage with guests from the moment of booking, providing instant customer service, answering queries, and offering additional services, which help in nurturing the customer's commitment to their stay. Furthermore, chatbots can upsell additional services effectively, such as spa appointments, dining reservations, or room upgrades, by seamlessly integrating recommendations into the conversation based on the guest's preferences and previous interactions. This not only enhances the guest experience by making personalized suggestions but also opens up new revenue opportunities for the hotel.
Integrating external data sources
Incorporating external data such as weather forecasts, flight cancellations, and economic forecasts can also help in predicting booking cancellations. AI can synthesize information from these diverse sources to make holistic predictions that account for a wide range of factors influencing customer behavior.
What is AutoML and how it enhances booking cancellation prediction
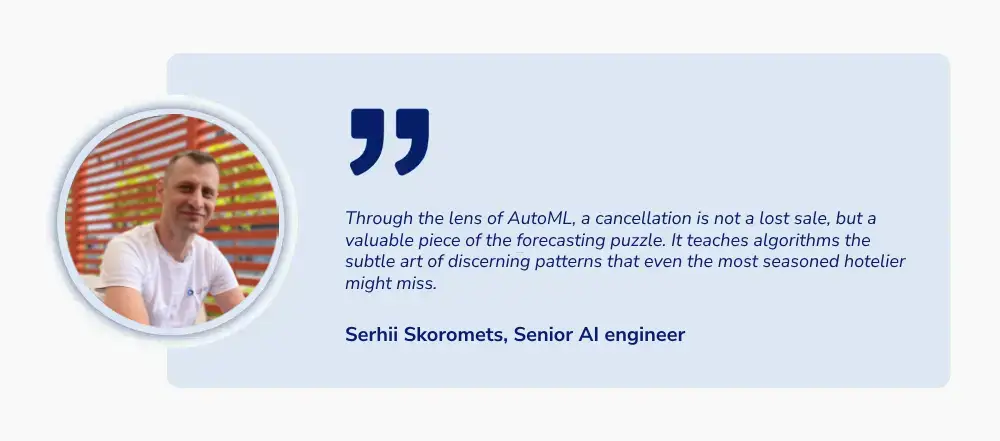
One of the standout technologies reshaping how hotels manage operational challenges is Automated Machine Learning (AutoML).
It provides hotels with a powerful tool to predict booking cancellations without needing deep technical knowledge. It simplifies analyzing vast amounts of data on booking patterns, seasonal trends, and other factors that influence cancellations.
Moreover, AutoML automates the process of choosing the best machine learning models, training these models with hotel data, and validating them to ensure they accurately predict cancellations. For example, consider a hotel chain encountering high cancellation rates during the off-season months. Traditionally, addressing these cancellations would require extensive data analysis. However, with AutoML, the hotel can automatically process historical booking data to identify patterns and causes of cancellations.
Armed with insights into these trends, the hotel might introduce flexible cancellation policies or special off-season promotions aimed at reducing cancellations and attracting more guests during this period.
Additionally, this capability means hotels can manage their inventory more effectively, reducing the financial impact of cancellations and enhancing guest experiences through improved service predictability and personalized offerings.
Implementing AutoML using Google Cloud Vertex AI to tackle cancellations
In our recent endeavor with a client facing the perennial challenge of booking cancellations, we choose to use AutoML through Google Cloud's Vertex AI to craft a robust proof of concept (PoC). This initiative aimed at harnessing the power of AutoML to not only predict but effectively reduce cancellations, presenting a practical application of this technology in the hospitality sector.
The following chapters represent a practical guide to the process.
1. Set up Google Cloud Platform (GCP)
The initial phase in harnessing Vertex AI for cancellation predictions begins with establishing a foundation on the Google Cloud Platform (GCP). This involves a few essential steps to ensure you have the necessary access and resources available:
- Begin by establishing your workspace on the Google Cloud Platform by either creating a new GCP account or logging into an existing one.
- After accessing the console, you'll need to create a new project, which will be the central hub for your machine-learning application.
- Name the project appropriately and connect it to a billing account since services like Vertex AI require billing to be enabled. Once your project is ready, navigate to the 'API & Services' to activate the Vertex AI API, allowing your project to interact with all the powerful tools and features Vertex AI has to offer.
2. Create datasets
With your GCP foundation set, the next step is data assembly. Here, you collect all relevant historical data that encompasses a range of aspects, such as booking logistics, customer histories, and room preferences – the more diverse, the better. A comprehensive dataset should include a variety of reservation details including:
- Booking information — Lead times, stay duration, arrival dates
- Guest profiles — Demographics, historical booking and cancellation records
- Room details — Preferences, types, and rates
- Ancillary requirements — Meal plans, special requests
Ensuring data quality is crucial; it involves cleaning data, handling missing values, normalizing varying scales, and encoding categorical variables for the machine's understanding.
3. Source data
Having cleaned your datasets, the next phase is to source this data into GCP. Upload the dataset to a GCP data storage solution like Google Cloud Storage, which will serve as the repository for your data, ready to be accessed and used by Vertex AI for model training.
4. Data check and analysis
Before diving into model training, inspect the uploaded data using the tools provided by Vertex AI or adjacent services on GCP. This preliminary analysis helps uncover insights about data distribution, quality, and potential issues that might affect model training. It also sets the stage for better-informed decision-making when selecting and tuning your machine-learning models.
5. Train model
Transitioning to model training, we can use Vertex AI to configure, initiate, and manage the training process. This includes choosing the right algorithm, defining the model's hyperparameters, and determining the computational resources needed. GCP guides you through this process, simplifying the complex task of training a predictive model at scale.
6. Model trained — let's check it
Once your model is trained, it's time to assess its performance. Evaluate critical metrics such as the model's accuracy and efficiency to ensure it meets your standards. Additionally, look into the model's feature import analysis, which sheds light on how different input variables influence the predictive outputs of the model.
Don’t forget to analyze which features are most influential in prediction decisions.
7. Deploy the model
With a trained and vetted model, the next step is deployment. GCP's Vertex AI offers different deployment options, ranging from real-time to batch predictions. Select the one that best matches your operational needs and deploy the model accordingly, setting it up to receive and process prediction requests.
8. Test and check results
Following deployment, we need to test the model rigorously with prediction requests to validate performance and reliability. During this phase, you may simulate various real-world scenarios, adjusting major parameters where necessary, and continue to refine the model based on test outcomes and local feature importance analyses.
You can adjust major parameters to simulate scenarios like ensuring no cancellations.
Check local feature importance to analyze the contribution of individual features in the prediction.
9. Simulate cancellation
Lastly, simulate a cancellation scenario to understand how the system responds and ensure the model’s robustness in predicting various outcomes.
10. Develop a user-friendly application
We wrapped up the project by developing the application for use in-house as the final step of our project. It turns complex data insights into a user-friendly tool that our clients can easily engage.
The benefits of such an application are multifold. This sort of app:
- Makes it easier to understand and act on data insights.
- Offers predictive insights for better, data-driven decisions.
- Tailored specifically to meet the unique needs of our client.
- Adjusts to real-time business conditions for up-to-date information.
- Embeds seamlessly into daily business operations without disrupting existing workflows.
- Designed for ease of use, requiring minimal training.
How will the system work in practice?
When a person responsible for managing bookings checks the system designed for managing booking cancellations, they see a specialized app that integrates real-time with Google Cloud's AutoML predictions.
Upon opening the app, the reservation manager is greeted with an intuitive dashboard that centralizes all the necessary information for efficient management. This dashboard dynamically updates to present the latest bookings, employing sophisticated algorithms to analyze and score each reservation based on its risk of cancellation. High-risk bookings are immediately noticeable, highlighted through visual cues such as color codes or icons, which draw the manager's attention without the need for sifting through individual records.
Potentially, predictive AI could also be integrated with built-in communication tools, the app facilitates direct engagement with guests, offering personalized incentives like upgrades or flexible booking terms to mitigate cancellation risks.
Where else this model can be applied
Predictive AI models initially designed for handling booking cancellations in hotels are not just limited to the hospitality industry. These techniques, powered by Machine Learning and AutoML, have broad applications across various sectors.
Of course, this doesn’t mean the model we have just described could be used for anything. Different industries and even different use cases within the same industry will require different datasets, formats, and models to learn. At the same time, it is comforting to know that AI/ML evolution has brought us far enough to be able to implement booking cancellation analysis and predict patterns.
Here is the breakdown of industries where booking cancellation prediction AI models are currently in high demand:
- Airlines: Just like in the hospitality industry, the airline industry can significantly reduce financial volatility by forecasting seat occupancy. Predictive systems can anticipate flight cancellations or no-shows, helping airlines manage overbooking and optimize load factors.
- Travel agencies: These agencies can utilize predictive analytics to offer personalized travel packages. By predicting potential cancellations, they can manage bookings effectively and propose alternative travel solutions to their customers, enhancing customer service and loyalty.
- Car rentals: Car rental companies can apply predictive models to estimate the likelihood of rental bookings being canceled. This data can be pivotal in managing fleet availability and pricing strategies to maximize utilization rates and revenue.
- Event management: Event planners and ticket sellers can use predictive systems to forecast attendance and cancellations for events. Such insights allow for better planning, resource allocation, and dynamic pricing to accommodate fluctuating demand.
- Healthcare: Hospitals and clinics can use predictive models to anticipate patient no-shows and last-minute appointment cancellations. This enables more efficient scheduling, reduces wait times, and ensures that critical resources are utilized effectively.
- Banking and insurance: Financial institutions can deploy predictive systems to anticipate customer churn and product cancellation rates. Understanding these patterns allows for more targeted marketing strategies and improves customer retention efforts.
- Retail: Retailers can benefit from predictive analytics by understanding potential shifts in consumer purchase behavior and seasonal demand. This information helps in stock optimization, minimizing overstocking or stockouts, and enhancing supply chain efficiency.
- Restaurants: Reservation no-shows in restaurants lead to loss of revenue and wastage of prepared food. Predictive systems help restaurants manage table inventory and staffing needs, possibly integrating dynamic booking policies to mitigate no-show impacts.
- Real estate: By predicting trends in housing markets, real estate agents can anticipate the ebb and flow of property demand, assess potential cancellations of viewings or transactions, and devise strategies to maintain a steady flow of business.
- E-Commerce: Predictive systems can be used to anticipate order cancellations or returns, helping e-commerce businesses to manage inventory more effectively and optimize their reverse logistics chain.
Conclusion
The journey to mitigate booking cancellations through the implementation of AutoML, specifically Google Cloud's Vertex AI, illuminates a compelling advancement within the hospitality industry. It's fascinating to see how even businesses with limited tech know-how can now tap into advanced predictive AI, which feels like democratizing superpowers.
This project not only tackled an age-old industry problem with modern technological innovations but also set a precedent for how data-driven approaches can significantly enhance operational efficiency and customer satisfaction.
The success of this PoC serves as a beacon for the broader hospitality sector, showcasing the tangible benefits of embracing artificial intelligence development services. Our AI team specializes in deploying a range of technologies including machine learning, natural language processing, image recognition, and predictive modeling, all geared towards making "AI superpowers" accessible to businesses of all sizes.